Project Code :TCMAPY536
Objective
The main objective of the project is to detect the type of the skin cancer using convolution neural networks.
Abstract
Skin cancer is one of the top three perilous
types of cancer caused by damaged DNA that can cause death. This damaged DNA
begins cells to grow uncontrollably and nowadays it is getting increased speedily.
There exist some researches for the computerized analysis of malignancy in skin
lesion images. However, analysis of these images is very challenging having
some troublesome factors like light reflections from the skin surface, variations
in color illumination, different shapes, and sizes of the lesions. As a result,
evidential automatic recognition of skin cancer is valuable to build up the
accuracy and proficiency of pathologists in the early stages. In this paper, we
propose a deep convolutional neural network (DCNN) model based on deep learning
approach for the accurate classification between benign and malignant skin
lesions. In preprocessing we firstly, apply filter or kernel to remove noise
and artifacts; secondly, normalize the input images and extract features that
help for accurate classification; and finally, data augmentation increases the
number of images that improves the accuracy of classification rate. To evaluate
the performance of our proposed, DCNN model is compared with some transfer
learning models such as AlexNet, ResNet, VGG-16, DenseNet, MobileNet, etc. The
ultimately we obtained training and testing accuracy respectively. The final
outcomes of our proposed DCNN model define it as more reliable and robust when
compared with existing transfer learning models.
NOTE: Without the concern of our team, please don't submit to the college. This Abstract varies based on student requirements.
Block Diagram
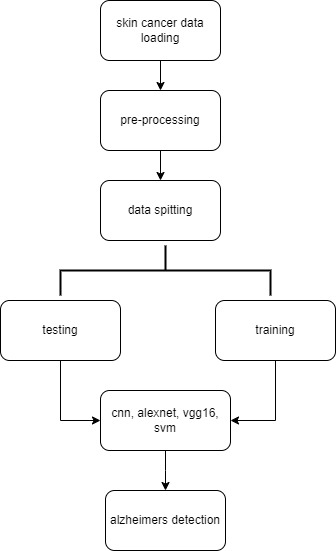
Specifications
Hardware:
- Operating
system: Windows 7 or 7+
- RAM: 8 GB
- Hard
disc or SSD: More than 500 GB
- Processor : Intel 3rd generation or high or Ryzen with 8
GB Ram
Software:
- Software’s: Python 3.6 or high version
- IDE : PyCharm.
- Libraries Used: Numpy, IO, OS, Flask, keras, pandas, tensorflow
Learning Outcomes
·
Hardware and software tools
·
Solution providing for real time problems
·
Working with team/individual
·
Work on creative ideas
- Testing techniques
- Error correction mechanisms
- What type of technology versions is used?
- Working of Tensor Flow
- Implementation of Deep Learning techniques
- Working of CNN algorithm
- Working of Transfer Learning methods
- Building of model creations
- Scope of project
- Applications of the project
- About Python language
- About Deep Learning Frameworks
- Use of Data Science